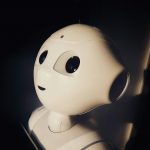
Access to psychological well being assist is just not equally distributed (Centre for Mental Health, 2020). Despite current authorities commitments to enhance the accessibility of psychological well being companies, variations nonetheless exist in sure inhabitants teams’ “ability to seek” and “ability to reach” companies (Lowther-Payne et al., 2023). Key obstacles embody experiences of – or anticipating experiences of – stigma, in addition to belief in psychological well being professionals (Lowther-Payne et al., 2023).
In a current paper, Habicht and colleagues (2024) recommend that there’s robust proof that digital instruments might assist overcome inequalities in treatment entry. The authors have been primarily referring to Limbic, a personalised synthetic intelligence (AI) enabled chatbot answer for self-referral. This personalised self-referral chatbot is seen to any particular person who visits the service’s web site and collects data required by the NHS Talking Therapies companies in addition to scientific data equivalent to the PHQ-9 and GAD-7. All knowledge are connected to a referral file inside the NHS Talking Therapies companies digital well being file – “to support the clinician providing high-quality, high-efficiency clinical assessment”.
So are chatbots the answer to inequalities in treatment entry? Within this weblog we take a better have a look at the proof behind Habicht and colleagues’ declare and ask the place this leaves us going ahead.
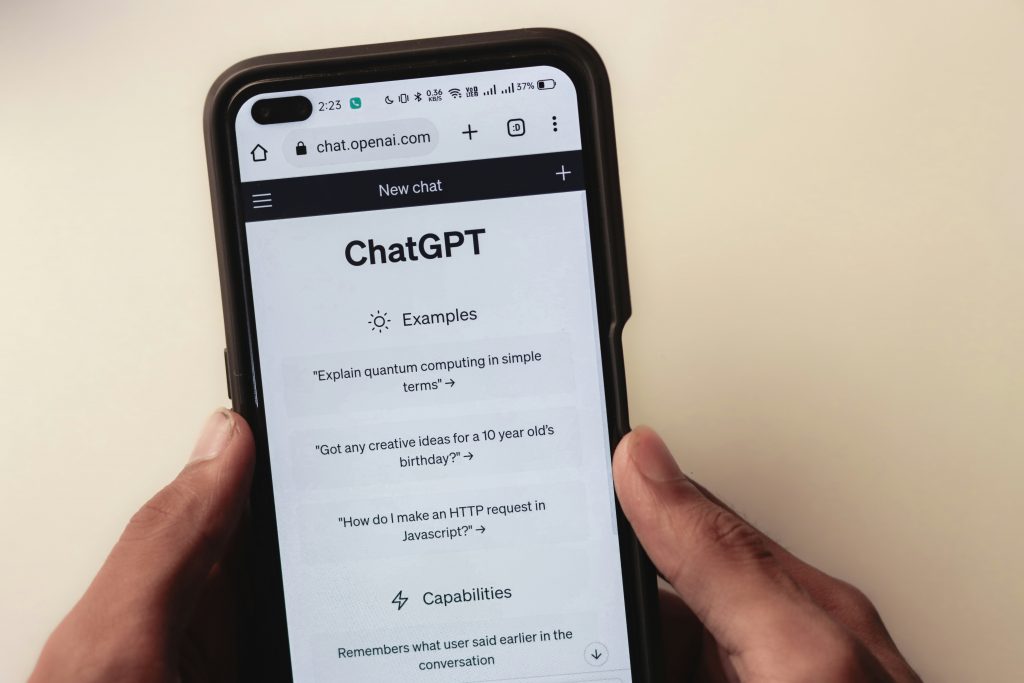
Are chatbots the answer to lowering inequalities in psychological well being treatment entry? Habicht and colleagues (2024) recommend they’re.
Methods
The authors performed an observational real-world research utilizing knowledge from 129,400 sufferers referred to 28 completely different NHS Talking Therapies companies throughout England. Fourteen of those companies carried out the self-referral chatbot and these have been matched with 14 companies who didn’t. The authors paid appreciable consideration to this matching and solely included management companies that used a web based type (reasonably than calling in to a service) as this was thought of the closest referral possibility to the chatbot. Other concerns included:
- Number of referrals at baseline
- Recovery charges
- Wait instances.
Analysis investigated 3 months earlier than adoption of the chatbot and three months after launch, and primarily centered on a rise in the variety of referrals. To disentangle the contribution of the AI and the common usability of the self-referral chatbot, a separate randomised managed between-subjects research with three arms straight in contrast the personalised chatbot with a normal webform and an interactive (however not AI-enabled) chatbot. To discover any potential mechanisms driving findings, the authors additionally employed a machine studying method – specifically Natural Language Processing (NLP) to analyse suggestions given by sufferers who used the personalised self-referral chatbot.
Results
Services that used the digital answer recognized elevated referrals. More particularly, these companies which used the personalised self-referral chatbot noticed a rise from 30,690 to 36,070 referrals (15%). Matched NHS Talking Therapies companies with an analogous variety of whole referrals in the pre-implementation interval noticed a smaller enhance from 30,425 to 32,240 referrals (6%).
Perhaps of larger significance, a bigger enhance was recognized for gender and ethnic minority teams:
- Referrals for people who recognized as nonbinary elevated by 179% in companies which utilised the chatbot; in contrast to a 5% lower in matched management companies.
- The variety of referrals from ethnic minority teams was additionally considerably greater when put next to White people: a 39% enhance for Asian and Asian British Groups was noticed, alongside a 40% enhance for Black and Black British people in companies utilizing the chatbot. This was considerably greater than the 8% and 4% seen in management companies.
Average wait instances have been additionally in contrast to tackle considerations that elevated referrals might lead to longer wait instances and worse outcomes. This revealed no important variations in wait instances between pre- and post-implementation intervals of the companies that used the chatbot and people who didn’t. Analysis of the variety of scientific assessments recommend that the chatbot didn’t have a detrimental impression on the variety of assessments performed.
So why is the chatbot growing referrals? And why is that this enhance bigger for some minority teams?
According to the authors, the utilization of the AI “for the personalization of empathetic responses and the customization of clinical questions have a critical role in improving user experience with digital self-referral formats”. Analysis of free textual content offered at the finish of the referral course of (n = 42,332) discovered 9 distinct themes:
- Four have been constructive:
- ‘Convenient’,
- ‘provided hope’,
- ‘self-realization’, and
- ‘human-free’
- Two have been impartial:
- ‘Needed specific support’ and
- ‘other neutral feedback’
- Three have been detrimental:
- ‘Expected support sooner’,
- ‘wanted urgent support’ and
- ‘other negative feedback’.
Individuals from gender minority teams talked about the absence of human involvement extra steadily than females and males. Individuals from Asian and Black ethnic teams talked about self-realization about the want for treatment greater than White people.
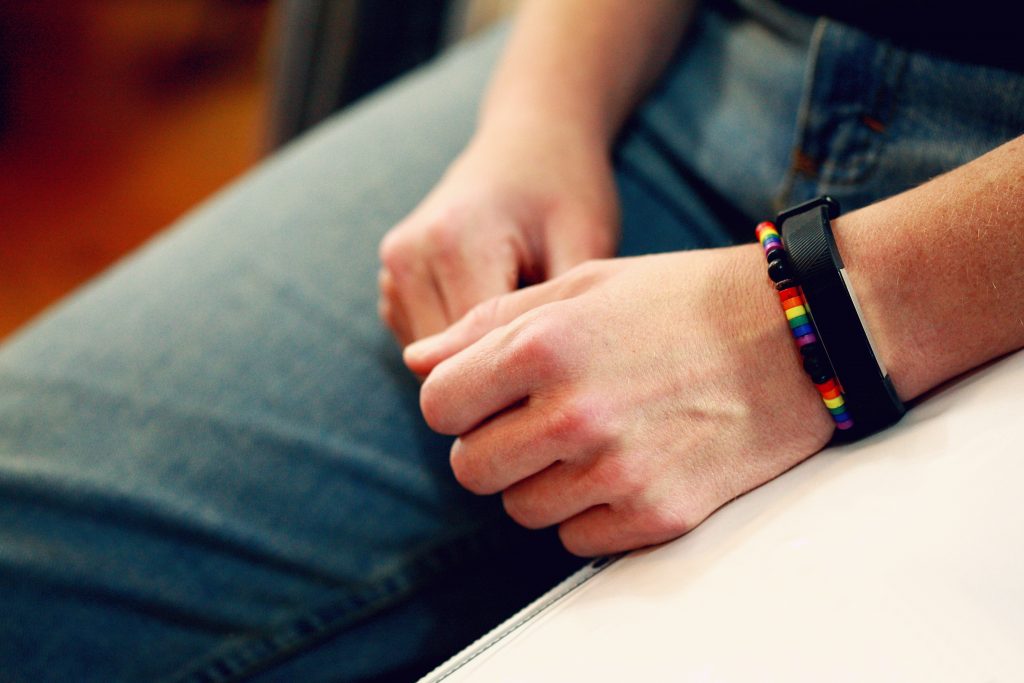
Services that used the chatbot recognized elevated referrals (15% enhance versus 6% enhance in management companies). This enhance was extra pronounced inside minority teams.
Conclusions
Findings strongly level towards the proven fact that personalised AI-enabled chatbots can enhance self-referrals to psychological well being companies with out negatively impacting wait instances or scientific assessments. Critically, the enhance in self-referrals is extra pronounced in minority teams, suggesting that this know-how might assist shut the accessibility hole to psychological well being treatment. The proven fact that ‘human-free’ was recognized as a constructive by individuals means that decreased stigma could also be an essential mechanism.
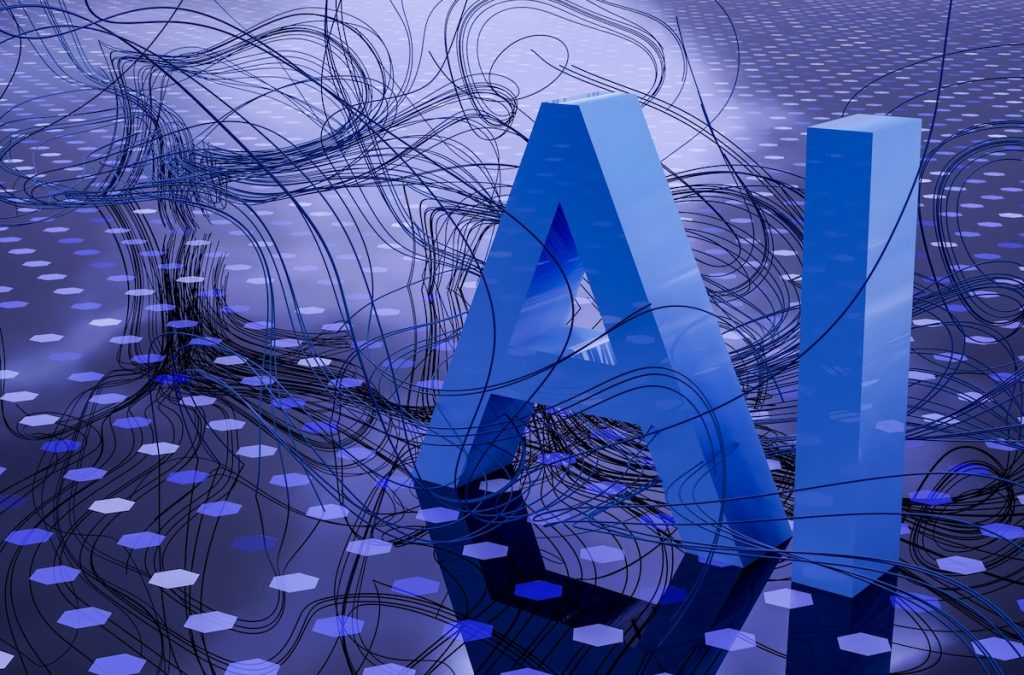
The proven fact that ‘human-free’ was recognized as a constructive by individuals means that decreased stigma could also be one cause why we see enchancment in the variety of entry.
Strengths and limitations
This is a well-considered research, with convincing findings. The authors have given appreciable thought to how companies ought to be matched and devised a collection of parallel analyses to management for confounders and disentangle attainable mechanisms, which will increase the reliability of the findings. At the similar time, this drive towards robustness has the potential to downplay a few of the complexities at play when contemplating inequalities to treatment entry.
This is probably finest seen in the NLP subject classification and dialogue of ‘potential mechanisms’. According to Leesen et al. (2019), qualitative researchers might discover NLP useful to assist their evaluation in two methods:
- First, if we carry out NLP after conventional evaluation, it permits us to consider the possible accuracy of codes created.
- Second, researchers can carry out NLP prior to open coding and use NLP outcomes to information creation of the codes. In this occasion, it’s advisable to pretest the proposed interview questions in opposition to NLP strategies as the type of a query impacts NLP’s skill to negotiate imprecise responses.
Habicht and colleagues’ method seems to straddle the two – first performing thematic evaluation on a pattern of the suggestions after which utilizing this in a supervised mannequin. Whilst the authors present an in depth dialogue of this analytical method, they provide much less by the use of justification. Do they take into account this arm to be qualitative analysis? Or is it merely that the evaluation was carried out on ‘qualitative free-text’?
Either means, it appears essential to word that features of the supervised NLP subject classification was carried out on textual content with a median entry size of 51 characters. That is roughly the size of this sentence. Whilst it might seem to be the query of ‘potential mechanisms’ has been answered, how we ask these questions issues.
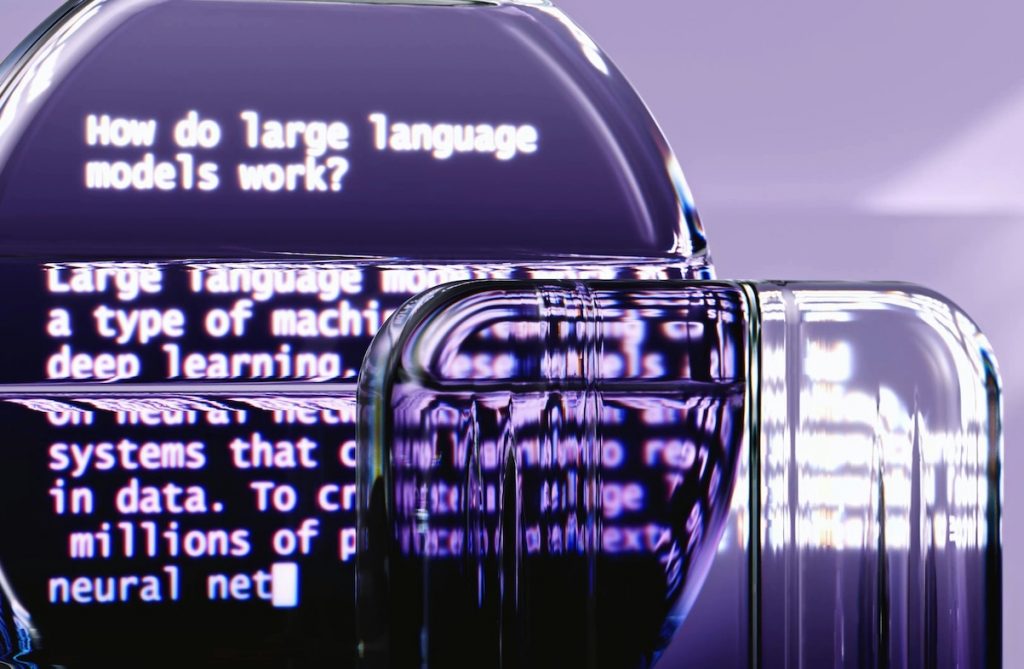
Whilst pure language processing clearly gives perception into the mechanisms underlying these findings, wealthy qualitative analysis appears obligatory if we’re to additional unravel these complexities.
Implications for observe
It is right here that we are able to return to the query of ‘where does this all leave us going forward’? Dr Niall Boyce from Wellcome requested an analogous query of the article in a current abstract:
An empathetic chatbot is preferable to filling in a type unaided, which is probably not the largest shock. It’s attainable that chatbots may help a extra various vary of individuals to entry companies…however what then? Would a “human free” therapist be secure, acceptable, and interesting as folks proceed their journey?
This is helpful in serving to body some preliminary ideas on implications.
First, the research does recommend that it’s greater than merely being preferable to filling in a type unaided. The authors straight examine the personalised self-referral chatbot with a normal webform and an interactive and user-friendly – however not AI-enabled – chatbot. Scores on the consumer expertise questionnaire have been greater for the self-referral chatbot than all different types, however there are some challenges right here (e.g., asking individuals to think about themselves in a self-referral state of affairs).
Second, we do want to proceed to ask how personalised AI-enabled chatbots can enhance self-referrals and why this enhance is extra pronounced inside minority teams. We additionally want to be mindful- as Andy Bell makes clear in a current weblog on this web site – that “mental health is made in communities, and that’s where mental health equality will flourish in the right conditions”. How do chatbots work with and in opposition to the significance of communities, for instance?
Third, it’s fascinating to word that the absence of human involvement was seen as a constructive by some – particularly as the literature seems equivocal on this level. For instance, a current assessment highlighted how one research discovered that sufferers most well-liked interplay with a chatbot reasonably than a human for his or her well being care, yet one more discovered that individuals report larger rapport with an actual skilled than with a rule-based chatbot. Somewhat equally, perceived realism of responses and pace of responses have been thought of variously as acceptable, too quick and too sluggish (Abd-Alrazaq et al., 2021). Within our personal analysis on expectations, individuals didn’t view chatbots as ‘human’ and have been involved by the concept that they might have human traits and traits. At different factors, being like a human was thought of in constructive phrases. The boundaries between being human/non-human and being like a human weren’t at all times clear throughout participant’s narratives, nor was there a secure sense of what was thought of fascinating.
Part of the cause why each the literature and our personal outcomes seem advanced is due to heterogeneity in what chatbots are and what they’re getting used for. Reviews will usually embody chatbots used throughout self-management, therapeutic functions, coaching, counselling screening and analysis. Within our personal research, chatbots have been being imagined as each a selected and generic know-how – for instance a chatbot for analysis in addition to a extra common ‘chatbot for mental health’ – main to a spread of traditions, norms and practices getting used to assemble expectations and understandings (cf. Borup et al., 2006).
This distinction between particular and generic could also be useful when enthusiastic about implications for observe right here. Returning to the paper into account, Habicht and colleagues do clarify that implications for observe relate to the use of a selected know-how – a personalised AI-enabled chatbot answer for self-referral. In this particular occasion, the absence of human involvement is seen by some as a constructive.
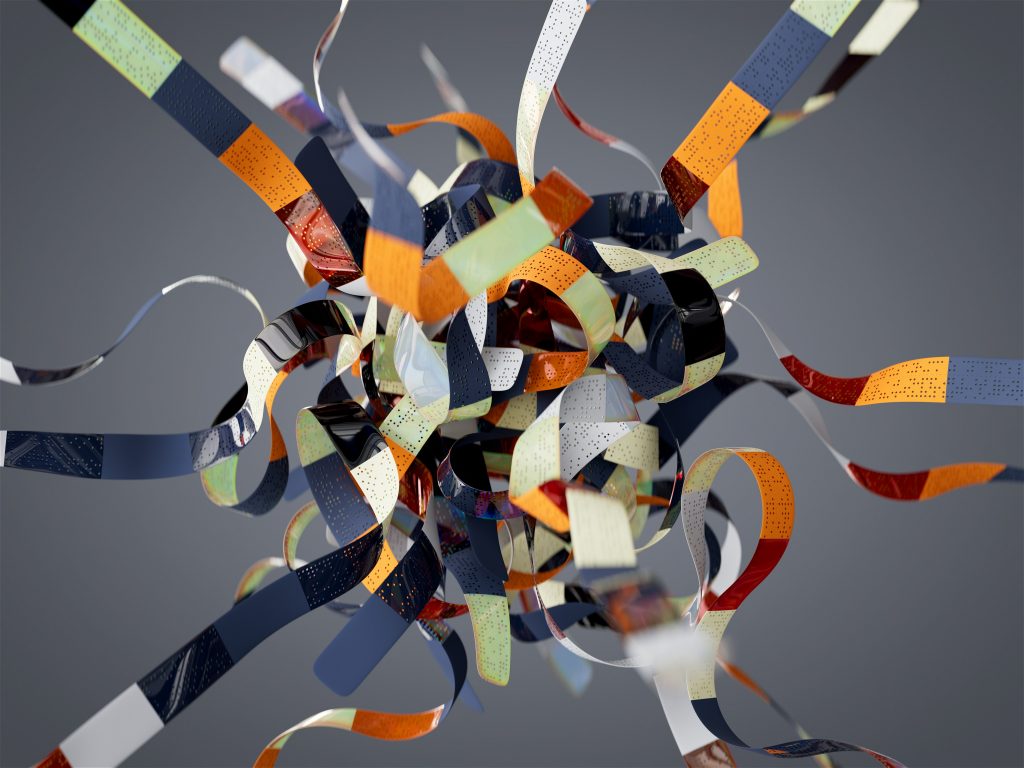
How do chatbots work with and in opposition to the significance of communities? This query, amongst many others, nonetheless wants to be addressed.
Statement of pursuits
Robert Meadows has not too long ago accomplished a British Academy funded undertaking titled: “Chatbots and the shaping of mental health recovery”. This work was carried out in collaboration with Professor Christine Hine.
Links
Primary paper
Habicht, J., Viswanathan, S., Carrington, B., Hauser, T. U., Harper, R., & Rollwage, M. (2024). Closing the accessibility hole to psychological well being treatment with a customized self-referral Chatbot. Nature Medicine, 1-8.
Other references
Abd-Alrazaq, A. A., Alajlani, M., Ali, N., Denecke, Ok., Bewick, B. M., & Househ, M. (2021). Perceptions and opinions of sufferers about psychological well being chatbots: scoping assessment. Journal of Medical Internet Research, 23(1), e17828.
Bell, A. (2024). Unjust: how inequality and psychological well being intertwine. The Mental Elf.
Borup, M., Brown, N., Konrad, Ok., & Van Lente, H. (2006). The sociology of expectations in science and know-how. Technology Analysis & Strategic Management, 18(3-4), 285-298.
Boyce, N. (2024). The weekly papers: Going human-free in psychological well being care; the dangers and advantages of legalising hashish; new enthusiastic about paranoia; greater physique temperatures and despair. Thought Formation.
Centre for Mental Health (2020). Mental Health Inequalities Factsheet. https://www.centreformentalhealth.org.uk/publications/mental-health-inequalities-factsheet/
Leeson, W., Resnick, A., Alexander, D., & Rovers, J. (2019). Natural language processing (NLP) in qualitative public well being analysis: a proof of idea research. International Journal of Qualitative Methods, 18.
Lowther-Payne, H. J., Ushakova, A., Beckwith, A., Liberty, C., Edge, R., & Lobban, F. (2023). Understanding inequalities in entry to grownup psychological well being companies in the UK: a scientific mapping assessment. BMC Health Services Research, 23(1), 1042.